Unlocking Efficiency with Machine Learning Labeling Service
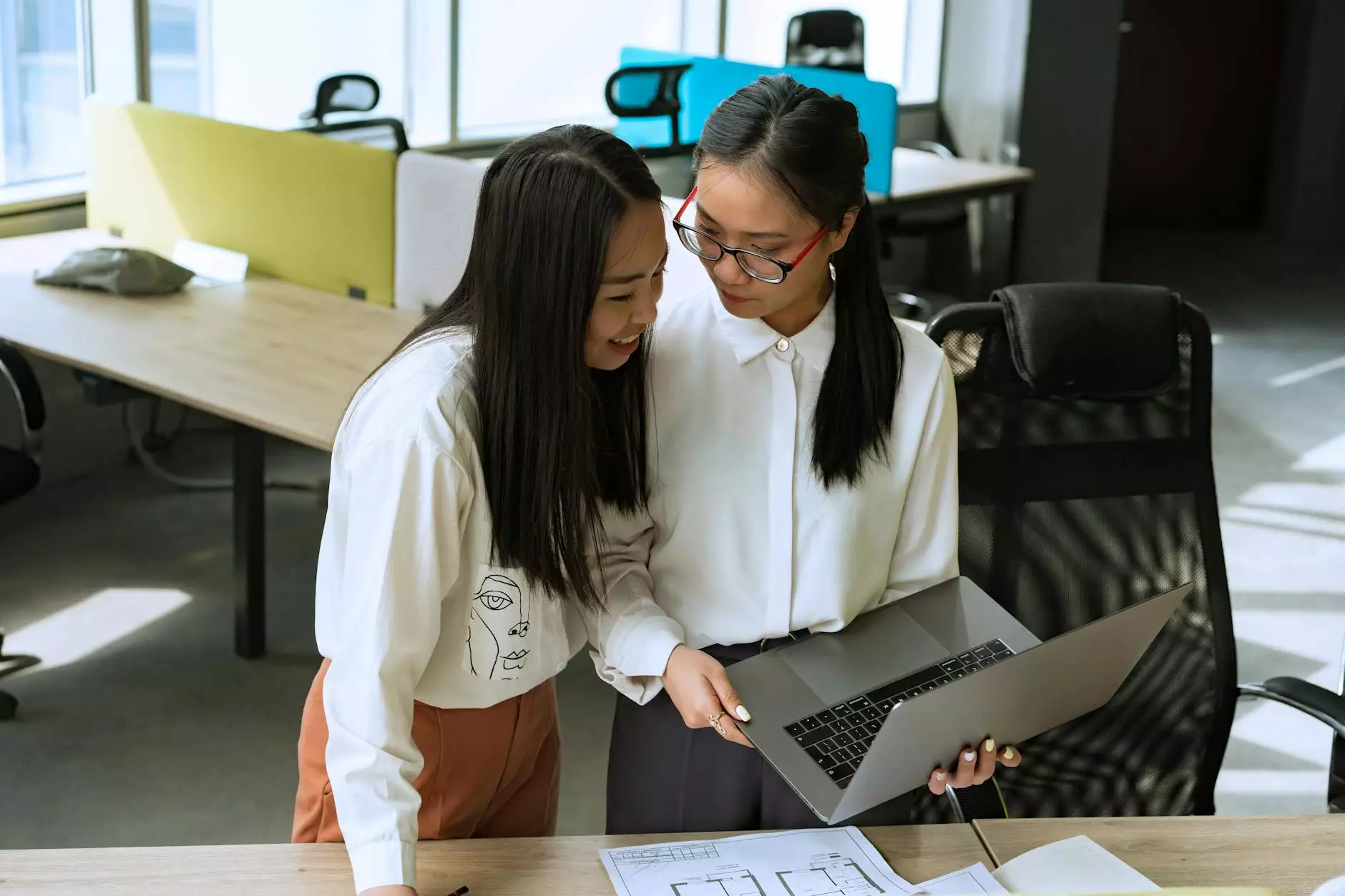
The digital age has ushered in an era where data has become one of the most valuable assets for businesses worldwide. To effectively harness this data, organizations are increasingly turning to machine learning labeling services as a pivotal component of their data annotation strategy. In this article, we will explore the significance, benefits, and implementation of these services, aiming to guide businesses towards achieving their operational goals more efficiently.
Understanding Machine Learning Labeling Services
Machine learning labeling services involve annotating datasets, allowing machine learning models to learn from the data more effectively. The primary goal of these services is to provide accurate labeled data that can be used to train algorithms for various applications, such as image recognition, natural language processing, and predictive analytics.
The Importance of Data Labeling
Data labeling is an essential part of the machine learning lifecycle. Here are some reasons why it's crucial:
- Enhances Model Accuracy: Properly labeled data improves the accuracy of machine learning models.
- Facilitates Automation: Accurate labeling allows businesses to automate processes that were once manual.
- Reduces Development Time: Well-annotated datasets mean quicker training times for models.
- Supports Scalability: As businesses grow, the number of datasets will increase, necessitating efficient labeling solutions.
Benefits of Using Machine Learning Labeling Services
Utilizing machine learning labeling services offers numerous benefits to businesses. Let’s delve into these advantages:
1. Accuracy and Consistency
One of the primary benefits of professional labeling services is the accuracy and consistency that trained annotators can provide. Machines learn best from clean, precise data. A well-executed labeling strategy can enhance the integrity of the dataset, thereby improving model performance significantly.
2. Time Efficiency
Labeling data manually can be a time-consuming process. Outsourcing this task to a machine learning labeling service can free up valuable time for your development team, allowing them to focus on what they do best: building and optimizing machine learning models.
3. Cost-Effectiveness
While there may be an upfront investment in labeling services, the long-term savings are significant. By avoiding the costs associated with errors and retraining models due to poorly labeled data, companies can save money and resources.
4. Access to Expertise
Hiring a dedicated labeling service provides access to a pool of experts in data annotation. These professionals are skilled in various domains, ensuring that your data is labeled according to industry standards and tailored to the specific needs of your projects.
5. Scalability
As businesses scale, so do their data needs. Machine learning labeling services can seamlessly adapt to larger volumes of data and more complex labeling tasks without compromising quality.
How to Choose the Right Machine Learning Labeling Service
Selecting the right machine learning labeling service is critical for the success of your data annotation projects. Here are some key factors to consider:
- Quality of Service: Look for reviews and case studies that demonstrate the quality of work.
- Experience: Choose a service with experience in your specific industry or type of data.
- Technology Integration: Ensure that the service can integrate smoothly with your existing technologies and workflows.
- Scalability: Confirm that the service can handle increasing volumes of labeling as your business grows.
- Support and Communication: Reliable communication is essential for successful collaboration.
Applications of Machine Learning Labeling Services
The scope of machine learning labeling services is vast, with applications spanning various sectors. Below we explore some of the key areas:
1. Retail and E-commerce
In the retail sector, image recognition models benefit significantly from accurate labeling of product images. Annotated data helps in developing systems that can recommend products or classify images efficiently, enhancing the customer experience.
2. Healthcare
In healthcare, machine learning models can analyze medical images, aiding in the early detection of diseases. Annotated data helps train algorithms that can differentiate between healthy and unhealthy tissues, thus supporting medical professionals in their diagnoses.
3. Autonomous Vehicles
For autonomous vehicle development, large quantities of labeled image and sensor data are crucial. This data helps machine learning algorithms understand the surrounding environment, identifying obstacles and making real-time decisions.
4. Natural Language Processing
In the realm of natural language processing, labeling text data assists in sentiment analysis, machine translation, and chatbot training. Accurate labeling allows for a better understanding of context and intent in communication.
Case Studies: Success with Machine Learning Labeling Services
To further illustrate the effectiveness of machine learning labeling services, let’s examine a few case studies that highlight real-world applications and successes:
Case Study 1: E-commerce Product Recognition
An e-commerce platform integrated a machine learning labeling service to improve their product recognition system. By providing detailed annotations on thousands of product images, the company was able to enhance their recommendation engine, resulting in a 25% increase in conversion rates.
Case Study 2: Medical Imaging Diagnostics
A healthcare provider partnered with a labeling service to annotate medical images for training a deep learning model. The results showed a dramatic improvement in diagnostic accuracy, which helped doctors reduce false negatives by 15%.
Case Study 3: Autonomous Vehicle Navigation
A major automotive company needed labeled datasets to train their autonomous vehicle systems. By applying machine learning labeling services, they successfully built a robust model that could navigate complex urban environments, achieving significant improvements in safety and efficiency.
Conclusion
In conclusion, machine learning labeling services represent a vital investment for businesses aiming to leverage the power of data effectively. By ensuring accurate, consistent, and scalable data annotation processes, organizations can significantly enhance the performance of their machine learning models, thereby gaining a competitive edge in their respective markets.
As data continues to proliferate in various forms, the importance of skilled labeling and careful data management cannot be overstated. For businesses eyeing growth and innovation, implementing a reliable machine learning labeling service is not just an option; it’s a strategic necessity.
For more information on implementing effective data annotation tools and platforms, visit keylabs.ai and explore how we can assist in your data journey.