The Essential Role of Semantic Segmentation Labeling Tools in Data Annotation
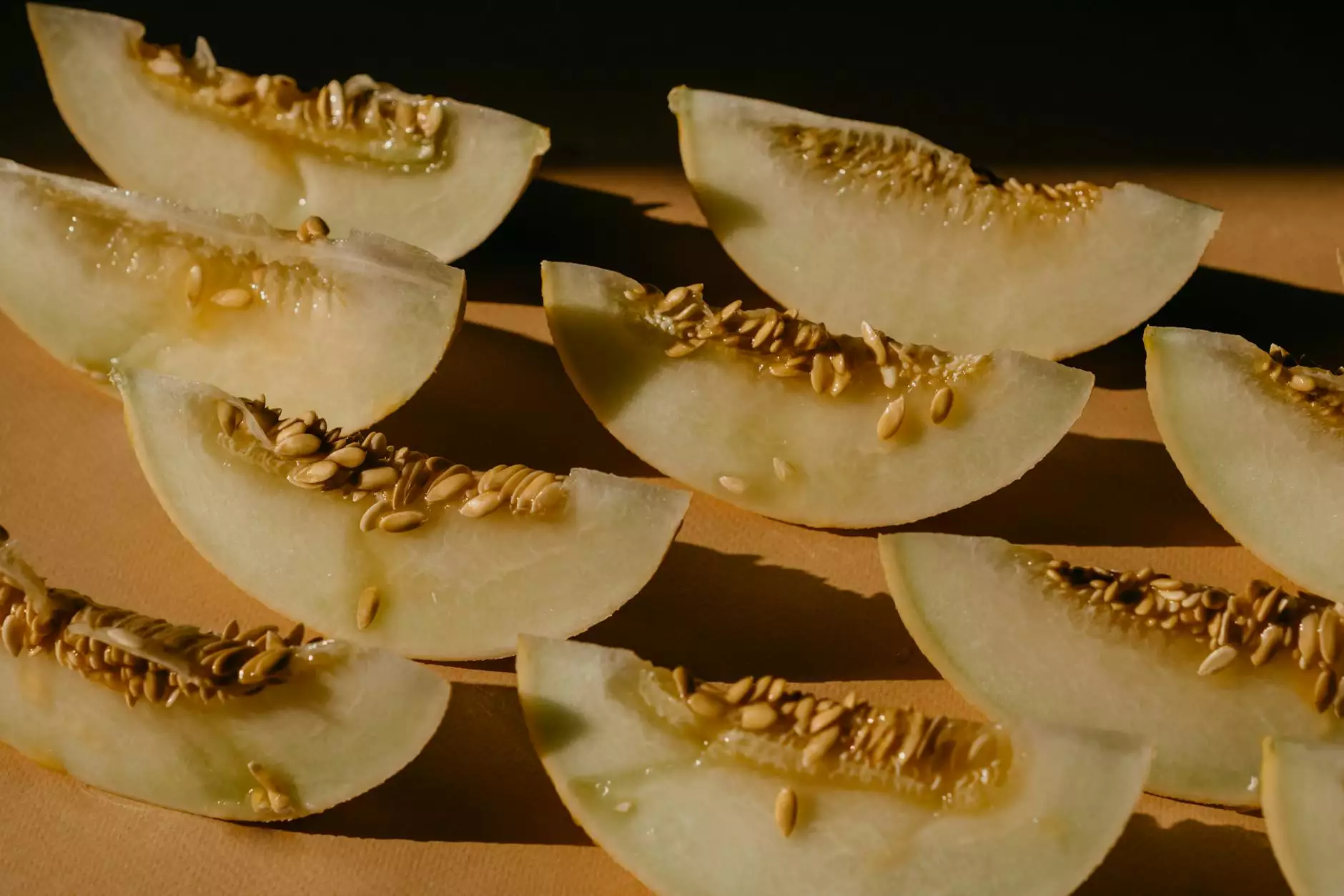
In the realm of artificial intelligence and machine learning, data annotation plays a vital role in training algorithms to understand and interpret vast datasets. Among various techniques, one that stands out in importance is the semantic segmentation labeling tool. This tool not only enhances the performance of models but also ensures that the data fed into algorithms is accurate and meaningful.
What is Semantic Segmentation?
Semantic segmentation is a process in computer vision that involves classifying each pixel of an image into a category or class. Unlike traditional image classification, which labels an entire image, semantic segmentation provides a more granular understanding of the image, making it essential for tasks where precision is crucial.
How Does Semantic Segmentation Work?
The fundamental goal of semantic segmentation is to enable the model to recognize the boundaries of objects and the spatial arrangement of various elements within an image. This is achieved through a series of deep learning models, particularly Convolutional Neural Networks (CNNs), which are trained on labeled datasets. The labeled datasets are produced using tools like semantic segmentation labeling tools, which aid in delineating the pixel-wise annotations for each class in the image.
Why Are Semantic Segmentation Labeling Tools Important?
Semantic segmentation labeling tools serve several crucial functions in the data annotation process:
- Precision Annotation: These tools allow for pixel-perfect annotation, which significantly improves the quality of datasets.
- Efficiency: By automating parts of the labeling process, these tools save valuable time and resources.
- Scalability: Organizations can easily scale their annotation efforts to meet the increasing demands of large datasets.
- User-Friendly Interfaces: Most tools come with intuitive interfaces that make it easy for users with varying degrees of technical expertise to produce high-quality annotations.
Key Features of Semantic Segmentation Labeling Tools
When selecting a semantic segmentation labeling tool, it is essential to consider several key features that enhance the annotation process:
1. Advanced Editing Capabilities
A powerful tool provides diverse editing options, allowing annotators to create complex shape outlines, refine edges, and manage multiple classes effectively. Look for features that support polygonal and manual editing, which are crucial for intricate tasks.
2. Real-Time Collaboration
Data annotation often requires teamwork. The best tools facilitate real-time collaboration, allowing multiple users to work on the same project simultaneously, streamlining the workflow and improving communication among team members.
3. Integration with AI Models
Many advanced semantic segmentation labeling tools offer integration capabilities with machine learning frameworks. This feature allows for a continual feedback loop where the model is trained, and results can be evaluated instantly to inform further iterations.
4. Support for Various Data Formats
Flexibility in dealing with different image formats (JPEG, PNG, TIFF) and output formats (COCO, VOC) ensures that organizations can use the tool without restructuring their existing data pipelines.
The Role of KeyLabs in Transforming Data Annotation
At KeyLabs, we harness the power of semantic segmentation labeling tools to provide cutting-edge data annotation solutions. Our platform is designed to meet the growing needs of AI development with features that enhance precision, efficiency, and productivity:
Industry-Leading Tools and Expertise
KeyLabs combines proprietary algorithms with user-centric design to develop tools that facilitate seamless workflow:
- Robust Annotation Capabilities: Our tools support complex tasks, including multi-class segmentation in challenging datasets.
- Automated Quality Control: Advanced techniques like AI-assisted annotation provide consistent quality by suggesting labels based on previously annotated samples.
- Scalable Solutions: Whether you need to annotate thousands of images or millions, our platform scales easily to meet your demands.
Focus on Customer Satisfaction
We work closely with clients to tailor our tools according to their unique requirements. With our dedicated customer support and comprehensive training resources, we ensure that teams can effectively utilize our semantic segmentation labeling tool, thus improving outcomes in their AI projects.
Applications of Semantic Segmentation in Various Industries
Understanding the relevance of semantic segmentation is crucial when discussing its applications across various sectors. Here are a few domains where semantic segmentation has made significant impacts:
1. Autonomous Vehicles
In the automotive sector, semantic segmentation is used to help self-driving cars understand their environment better. By accurately segmenting different elements like roads, pedestrians, and obstacles, these vehicles can make informed decisions, enhancing safety and efficiency.
2. Healthcare Imaging
In medical imaging, semantic segmentation aids in identifying and demarcating regions of interest in imaging scans, such as tumors. This precise identification allows for improved diagnostics and treatment planning.
3. Agricultural Technology
In agriculture, semantic segmentation helps in crop monitoring, disease detection, and yield prediction by analyzing aerial images captured by drones or satellites. This data-driven approach enhances productivity and resource management.
4. Retail and E-Commerce
Semantic segmentation tools are used in retail to enhance customer experiences through smart recommendations and dynamic inventory management. By analyzing visual data, retailers can optimize their layout and marketing strategies.
Challenges in Data Annotation
Despite the advantages of semantic segmentation labeling tools, there are challenges associated with data annotation:
1. Complexity of the Data
Some datasets may contain intricate images with overlapping objects or varying lighting conditions, making segmentation challenging. It requires skilled annotators and advanced tools with high accuracy.
2. Time-Consuming Process
Annotating large datasets can be a labor-intensive process, which can be mitigated through automation but may still require human oversight for quality assurance.
3. Quality Control
Ensuring the quality of annotated data is crucial. Tools that offer built-in quality assurance features are beneficial, but regular audits and feedback loops are also necessary.
The Future of Semantic Segmentation Labeling Tools
As we look towards the future, the development of semantic segmentation labeling tools will continue to evolve, driven by advancements in AI and deeper integration with machine learning workflows. The following trends are likely to shape the future landscape:
1. Increased Automation
With the rise of AI-assisted tools, the degree of automation in data annotation will likely increase. This change will lead to faster turnaround times and enable teams to focus more on strategic tasks rather than routine labeling chores.
2. Enhanced Customizability
Tools will become more customizable, allowing users to tailor features according to their specific needs, making them suitable for a wider range of applications.
3. Integration with Big Data Analytics
The synthesis of semantic segmentation tools with big data analytics will allow organizations to extract deeper insights from annotated datasets, enhancing their decision-making processes.
Conclusion
In today's rapidly evolving landscape of artificial intelligence and machine learning, the importance of effective data annotation cannot be overstated. The semantic segmentation labeling tool has emerged as a cornerstone technology that not only streamlines the annotation process but also boosts the performance of trained models. With solutions like those offered by KeyLabs.ai, organizations can leverage advanced annotation tools to propel their AI initiatives forward, ensuring they remain competitive in their respective fields.
By embracing cutting-edge technologies and methodologies in data annotation, businesses can unlock new potentials and drive innovation, ultimately leading to success in the data-driven world.