The Ultimate Guide to Data Labelling Tools for Businesses
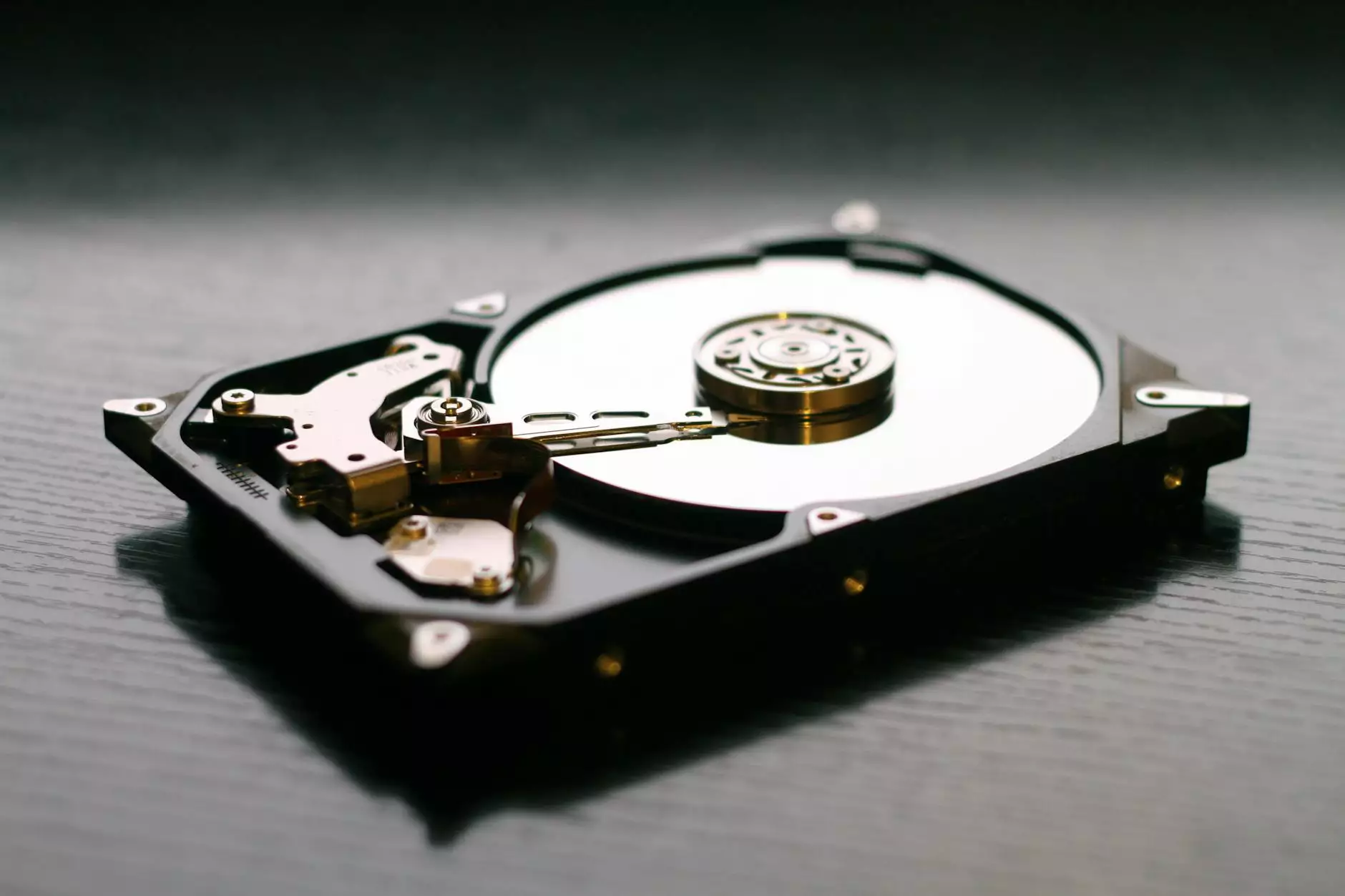
In the rapidly evolving world of technology, data labeling tools have emerged as crucial elements that fuel advancements in artificial intelligence (AI) and machine learning (ML). In this comprehensive article, we delve into what data labeling tools are, their significance in business operations, and how they can help companies like Keymakr optimize their processes.
What is a Data Labelling Tool?
A data labeling tool is a software application that helps organizations categorize and annotate large volumes of data, making it easier to train machine learning models. By providing structured training data, businesses can enhance the accuracy and performance of their AI systems.
Types of Data Labelling
- Image Labelling: This involves annotating images for computer vision applications, such as object detection, facial recognition, and image segmentation.
- Text Labelling: Common in natural language processing (NLP), text labeling includes sentiment analysis, named entity recognition, and text categorization.
- Audio Labelling: Audio data is labelled to facilitate speech recognition, audio classification, and more.
- Video Labelling: Involves annotating video data frames for applications like activity recognition and video classification.
Why Are Data Labelling Tools Essential for Businesses?
Data labelling tools are indispensable for organizations aiming to leverage AI and ML technologies. Here are several reasons why they are vital:
1. Enhanced Data Quality
High-quality labeled data is the backbone of effective machine learning models. Utilizing a data labeling tool ensures that the data is accurately labeled, reducing errors and improving the reliability of outcomes.
2. Accelerated Model Training
Data labeling can be a time-consuming process. A well-designed tool streamlines this workflow, allowing businesses to label large datasets quickly and efficiently, which speeds up the model training process.
3. Cost-Effectiveness
By automating parts of the labeling process, organizations can significantly reduce labor costs associated with manual data annotation. The return on investment (ROI) from implementing a data labeling tool can be substantial.
4. Scalability
As businesses grow, their data volume increases dramatically. Data labeling tools are designed to scale alongside organizations, ensuring that they can handle increased workloads without a hitch.
Choosing the Right Data Labelling Tool
Given the multitude of options available, choosing the right data labeling tool for your business can be challenging. Here are some factors to consider:
1. Ease of Use
The tool should have an intuitive interface that allows team members to start annotating with minimal training. A user-friendly experience is essential for maximizing productivity.
2. Supported Data Types
Ensure that the tool supports the specific data formats your business needs, whether that be images, text, audio, or video. The more versatile the tool, the better it can adapt to your requirements.
3. Collaboration Features
In a team setting, collaboration is key. Look for tools that promote teamwork through features such as real-time editing, comment sections, and task assignments.
4. Integration Capabilities
Consider whether the tool integrates seamlessly with your existing software infrastructure, including data storage solutions and machine learning platforms.
Benefits of Using Data Labelling Tools in Various Industries
Data labeling tools are not just for tech companies. They provide immense value across various sectors.
1. Healthcare
In healthcare, data labeling is crucial for diagnosing diseases through medical imaging and managing patient data. Labeled data can improve AI algorithms designed for predicting patient outcomes or classifying medical conditions.
2. Automotive
The automotive industry utilizes data labeling tools extensively for training autonomous vehicles. By labeling sensor data, companies can enhance the safety and reliability of self-driving technologies.
3. Retail
Retailers leverage data labeling for analyzing customer behavior, improving inventory management, and personalizing shopping experiences based on consumer data.
4. Finance
In finance, data labeling aids in fraud detection by identifying and classifying suspicious transactions through historical data analysis.
Steps to Implement a Data Labelling Tool in Your Business
Implementing a data labeling tool in your business requires strategic planning. Here’s how to do it effectively:
1. Identify Your Use Case
Understand the specific problems you are trying to solve with data labeling. Whether it’s for customer segmentation or predictive analytics, having a clear objective is crucial.
2. Select the Right Tool
Based on the considerations mentioned earlier, choose a tool that aligns with your business’s needs and objectives.
3. Train Your Team
Provide adequate training to your team to ensure they can efficiently use the tool. This can involve workshops, user manuals, and online resources.
4. Start with a Pilot Project
Before fully rolling out the tool, conduct a pilot project to gauge its effectiveness. Evaluate user feedback and performance metrics to make necessary adjustments.
5. Monitor and Optimize
Continually monitor the data labeling quality and the tool’s performance. Use insights to optimize your processes further, ensuring that your machine learning models remain accurate and reliable.
Challenges in Data Labelling and How to Overcome Them
While data labeling tools offer significant benefits, challenges exist. Here are some common issues and how to address them:
1. Annotation Errors
Inaccurate labeling can seriously affect ML model performance. To mitigate this, implement quality control processes that include multiple reviews for accuracy.
2. High Volume of Data
The sheer volume of data requiring labeling can be daunting. Consider leveraging a combination of automated tools and human annotators to balance efficiency and quality.
3. Changing Requirements
Business needs can evolve, leading to changes in labeling requirements. Ensure your chosen tool is flexible and adaptable to new criteria and projects.
Conclusion
The relevance of data labeling tools in today's business landscape cannot be overstated. By utilizing these tools, companies not only streamline their data processing capabilities but also unlock the full potential of AI and ML to drive innovation. Embracing the right data labeling tool can lead to better decision-making, enhanced customer experiences, and ultimately, improved business outcomes.
For organizations looking to stay ahead in their industry, investing in a robust data labelling tool will serve as a cornerstone in their journey towards digital transformation. As machine learning continues to shape the future of business, ensuring your data is well-labeled and ready for analysis will keep your company at the forefront of innovation.